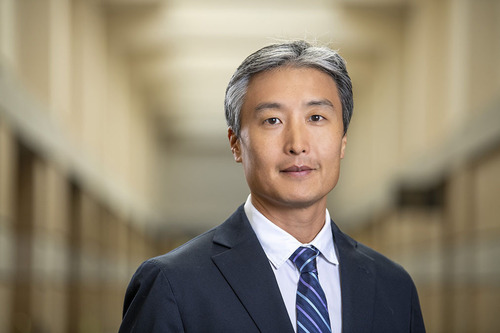
More than two-thirds of the world’s population is expected to live in cities by 2050, according to the United Nations. As urbanization advances around the globe, researchers at the University of Notre Dame and Stanford University said the quality of the urban physical environment will become increasingly critical to human well-being and to sustainable development initiatives.
However, measuring and tracking the quality of an urban environment, its evolution and its spatial disparities is difficult due to the amount of on-the-ground data needed to capture these patterns. To address the issue, Yong Suk Lee, assistant professor of technology, economy and global affairs in the Keough School of Global Affairs at the University of Notre Dame, and Andrea Vallebueno from Stanford University used machine learning to develop a scalable method to measure urban decay at a spatially granular level over time.
Their findings were recently published in Scientific Reports.
“As the world urbanizes, urban planners and policymakers need to make sure urban design and policies adequately address critical issues such as infrastructure and transportation improvements, poverty and the health and safety of urbanites, as well as the increasing inequality within and across cities,” Lee said. “Using machine learning to recognize patterns of neighborhood development and urban inequality, we can help urban planners and policymakers better understand the deterioration of urban space and its importance in future planning.”
Traditionally, the measurement of urban quality and quality of life in urban spaces has used sociodemographic and economic characteristics such as crime rates and income levels, survey data of urbanites’ perception and valued attributes of the urban environment, or image datasets describing the urban space and its socioeconomic qualities. The growing availability of street view images presents new prospects in identifying urban features, Lee said, but the reliability and consistency of these methods across different locations and time remains largely unexplored.
In their study, Lee and Vallebueno used the YOLOv5 model (a form of artificial intelligence that can detect objects) to detect eight object classes that indicate urban decay or contribute to an unsightly urban space — things like potholes, graffiti, garbage, tents, barred or broken windows, discolored or dilapidated façades, weeds and utility markings. They focused on three cities: San Francisco, Mexico City and South Bend, Indiana. They chose neighborhoods in these cities based on factors including urban diversity, stages of urban decay and the authors’ familiarity with the cities.
Using comparative data, they evaluated their method in three contexts: homelessness in the Tenderloin District of San Francisco between 2009 and 2021, a set of small-scale housing projects carried out in 2017 through 2019 in a subset of Mexico City neighborhoods, and the western neighborhoods of South Bend in the 2011 through 2019 period — a part of the city that had been declining for decades but also saw urban revival initiatives.
Researchers found that the trained model could adequately detect the objects it sought across different cities and neighborhoods, and did especially well where there are denser populations, such as San Francisco.
For instance, the maps allowed researchers to assess the temporal and geographic variation in homelessness in the San Francisco area, an issue that has grown over the years.
The model struggled in the more suburban area of South Bend, according to Lee, demonstrating a need to tweak the model and the types of objects identified in less dense populations. In addition, the researchers found there is still a risk for bias that should be addressed.
“Our findings indicate that trained models such as ours are capable of detecting the incidences of decay across different neighborhoods and cities, highlighting the potential of this approach to be scaled in order to track urban quality and change for urban centers across the U.S. and cities in other countries where street view imagery is available,” he said.
Lee said the model has potential to provide valuable information using data that can be collected in a more efficient way compared to using coarser, traditional economic data sources, and that it could be a valuable and timely tool for the government, nongovernmental organizations and the public.
“We found that our approach can employ machine learning to effectively track urban quality and change across multiple cities and urban areas,” Lee said. “This type of data could then be used to inform urban policy and planning and the social issues that are impacted by urbanization, including homelessness.”
Contact: Tracy DeStazio, associate director of media relations, 574-631-9958 or tdestazi@nd.edu
Originally published by April Toler at keough.nd.edu on Oct. 19.